第一届CCF农业知识智能工程国际学术论坛(AKIE2023)即将于6月10日正式开启,随着每个特邀报告内容的陆续敲定,参会者热情持续高涨。本文发布完整大会日程,供参会者参考。
今天,备受关注的大会日程最新版发布,看看都有哪些值得期待的精彩内容吧。
2023年6月10日 (微重B座103报告厅) |
|
8:00-8:15 |
开幕式(校领导致辞) |
8:15-8:30 |
大会合影 |
8:30-9:15 |
何克清,武汉大学计算机学院教授 报告题目:高层软构体具身交互认知及其群体心智的研究 |
9:15-9:45 |
邓水光,浙江大学计算机科学与技术学院教授 报告题目:云边协同计算与智能 |
9:45-10:00 |
休息 |
10:00-10:30 |
赵瑞雪,中国农科院农业信息研究所副所长 报告题目:“数据+AI”驱动的农业智能知识服务发展思考 |
10:30-11:00 |
章文,华中农业大学信息学院教授 报告题目:人工知识智能大健康 |
11:00-11:30 |
Michael Bewong,澳大利亚查理斯特大学副教授 报告题目:Machine Learning in Precision |
11:30-14:00 |
午餐 |
14:00-14:45 |
刘经南,中国工程院院士,武汉大学教授 报告题目:时空智能赋能智慧农业 |
14:45-15:15 |
叶育鑫,吉林大学计算机科学与技术学院教授 报告题目:从作物表型数据到知识图谱 |
15:15-15:45 |
温长吉,吉林农业大学信息技术学院副院长 报告题目:视觉解译关键技术研究及农业应用 |
15:45-16:15 |
冯在文,华中农业大学信息学院副教授 报告题目:农业知识智能服务 |
16:15-16:30 |
休息 |
16:30-17:00 |
段玉聪,海南大学计算机科学与技术学院教授 报告题目:从数据到智慧:意图驱动的AGI-AIGC-GPT大模型的认知测评 |
17:00-17:30 |
Pierre Larmande,法国发展研究所研究员 报告题目;Data Integration and Knowledge Extraction in Agronomy: Examples |
17:30-18:00 |
Claire Nedellec,法国国家农业食品与环境研究院研究员 报告题目:Making textual information and |
18:15 |
晚餐 |
院士领衔 链接行业高端视野
资深专家云集 突围行业发展瓶颈
CCF服务计算专委、武汉大学何克清教授
题目:高层软构体具身交互认知及其群体心智的研究
秉承“机器具身(embodied)交互智能”、“机器的生命观”,揭示并阐明在面向信息的低层软构体(数据、算法、语言、信息系统等)之上,面向知识和智能的高层软构体,是向下知识化共享管理跨模态多样异构的大数据资源、向上凝练融合沉淀交互智能增值增量为共性目标,其内涵,如元数据、本体、元模型/本体元模型、可互操作的信息系统模型,及支撑交互控制与协调自复用自生长的泛在互操作系统工具平台等。重点阐述了引导交互认知的 RGPS 需求知识元模型方法,交互记忆与学习的知识语义互操作性元模型框架 MFI 机制,及其 ISO/IEC 19763 标准系列,以及交互认知应用的群体心智。本研究与实践,有 突破了现有 I AI 软件 和信息系统 软件 的四大局限:①突破现有信息系统软件的价值局限:从存值、存量,到通过具身交互控制与生态环境协调演变自生长,实现“异构集成”1+1>2 的增值增量。②突破交互认知复杂系统混沌易发的局限:阐明了交互认知赖需(求)秩序为生的科学问题,创造了 RGPS 需求知识元模型框架机理。③突破现有AI软件忽视记忆的局限:强调记忆智能优先于计算智能;发现了模型交互记忆的类脑机理 LTP 特性,主导研制了长时记忆的MFI(O-RGPS)国际标准系列—ISO/IEC 19763-3,5,7,8,9。④突破现有软件模型的局限:从强调模型的复用与转换,到强调模型的撕裂、碎片化及演化,为按需选择跨域高层软构体模型的交互认知,实现“异构集成”、自复用、自繁衍生长,提供了可预测的方法。
CCF服务计算专委常务委员、国家杰出青年基金获得者、浙江大学邓水光教授
题目:云边协同计算与智能
边缘计算将云的计算和存储能力下沉到网络边缘,向用户提供本地化服务,降低核心网压力,是对云计算架构的补充和延伸。云边协同充分发挥边缘计算与云计算的优势,是解决复杂应用场景的重要计算范式。云边协同智能是人工智能、边缘计算与云计算的有机融合,可有效解决人工智能最后一公里问题。本报告从计算范式的演变出发,介绍了边缘计算、云边协同、边缘智能等关键技术的前沿热点,并重点分享了在云边协同、边缘智能等方面的研究进展和阶段性成果。
中国农业科学院农业信息研究所 赵瑞雪研究员
题目:“数据+AI”驱动的农业智能知识服务发展思考
分析农业智能知识服务的关键要素和主要场景,总结在农业信息智能感知、跨媒体农业知识组织和智能服务的研究进展与实践,探索“数据+AI”驱动的知识服务发展新趋势。
CCF生物信息学专委会专委、华中农业大学教授章文
题目:人工知识智能大健康
随着我国生活水平的提高,人民群众对于健康的要求越来越高。人工智能是革命性的科技力量,能够有力推动健康领域发展,发展面向大健康的人工智能方法成为迫切需要,是实现面向人民生命健康的重要途径。我们将探讨疾病治疗、药物发现等大健康领域的人工智能技术的发展历史、现状和未来,介绍可应用于大健康领域的图表示学习模型、深度学习模型、自监督学习模型等。最后,以治未病为例,展望人工智能技术如何在其中发挥重要作用。
澳大利亚查理斯特大学、Michael Bewong教授
题目:Machine Learning in Precision Agriculture
Precision agriculture represents the new age of conventional agriculture. This is made possible by the advancement of various modern technologies such as the internet of things and distributed sensor networks. The unparalleled potential for data collection and analytics has resulted in an increase in multi-disciplinary research within machine learning and agriculture. In this talk, we will canvass the applied machine learning research in agriculture by revisiting academic literature published in the last two decades. We will identify and discuss some of the key issues relating to methodological approaches of machine learning in agriculture, and data management issues such as data sharing, and data quality. The talk will also highlight the impact of these challenges to knowledge discovery from agricultural data, and propose some mitigation strategies as well as future research directions. Finally, the talk will be concluded by introducing a newly developed framework, by Charles Sturt, aimed at tackling one of the key issues in agricultural data, namely data integration. The framework called Semi-Automatic Hybrid Schema Matching (SAHM) was developed to address the problem of integrating disparate and distributed vegetation data within the Australian context.
CCF数字农业分会执行委员、吉林大学叶育鑫教授
题目:从作物表型数据到知识图谱
表型组学是在基因组水平上系统研究某一生物或细胞在不同环境下所有表型的科学。植物表型无损获取方法的发展以及大规模自动化高通量表型获取设施的建立,解决了表型数据获取效能不足以及与基因数据、环境数据获取效能不匹配的问题,但同时也给表型数据管理带来新的挑战。本次报告将介绍国内外作物表型大数据知识图谱化的最新进展,并进一步提出“表型数据图谱化”和“图谱知识数据化”的表型数据迭代增量发展模式,为作物表型组学数据管理提供新思路。
CCF数字农业分会常务委员、吉林农业大学副教授温长吉
题目:视觉解译关键技术研究及农业应用
机器视觉解译技术在动植物表型、病虫害监测等方面的应用正逐渐成为农业应用的研究热点,但是相关领域的场景应用仍然面临技术挑战。视觉解译技术包括图像分割、目标检测、图像分类等技术,可实现对大规模图像数据的自动处理和分析。依据场景任务驱动,利用视觉解译技术实现对动植物的各种表型性状进行量化表示和描述,为农业生产数字化建模,服务科学种植、农业管理等提供技术支持。
CCF服务计算专委、华中农业大学副教授冯在文
题目:农业知识智能服务
目前,我国农业智能化软件技术有效供给不足,大力发展以知识工程技术为核心的农业智能化软件,对大幅度提高农业资源利用率和生产效率,实现农业高质量发展具有重要作用。报告将介绍:(1)在农业知识建模领域的顶层链接本体元建模方法;(2)在农业知识抽取领域的知识与数据双轮驱动的结构化数据语义抽取方法;(3)在农业知识融合领域基于图函数依赖的实体解析与缺失值补全方法;(4)在农业知识更新领域的主动元学习知识更新方法;(5)在农业知识服务领域的知识图谱增强的农业GPT等一系列原创性成果。
CCF服务计算专委、海南大学段玉聪教授
题目:从数据到智慧:意图驱动的AGI-AIGC-GPT大模型的认知测评
本报告涉及从数据到智慧的过程,并以意图驱动的AGI-AIGC-GPT大模型的认知测评为重点。DIKWP模型作为一个理论框架,解释了数据、信息、知识和智慧之间的转化过程,并强调了意图在其中的核心作用。首先介绍了DIKWP模型的元素,包括数据、信息、知识、智慧和意图。数据代表原始的、未经处理的事实和数字,而信息是在特定上下文中被解释和理解的数据,提供了意义和洞察力。知识是全面理解的信息,并应用于决策过程,包括对模式和趋势的识别。智慧是基于知识、价值观、洞察力和经验做出明智决策和判断的能力。而意图则是驱动数据、信息、知识和智慧之间交互和转化的目标。聚焦于AGI-AIGC-GPT大模型的认知测评,这是一个以意图驱动的模型为核心的评估过程。该模型结合了人工通用智能(AGI)、人工意图生成与理解(AIGC)以及生成式预训练模型(GPT)的概念和技术。总结了意图驱动的AGI-AIGC-GPT大模型的认知测评的重要性和潜在应用。AGI-AIGC-GPT大模型的DIWKP认知测评方法提供了一种有效的评估工具。通过测评,我们能够评估这个大型模型在认知任务上的表现和效能,对于推动人工智能领域的发展和应用具有重要意义。
注:AGI指的是人工通用智能(Artificial General Intelligence),AIGC指的是人工意图生成与理解(Artificial Intention
Generation and Comprehension),GPT指的是生成式预训练模型(Generative Pre-trained Transformer)。
法国发展研究所研究员Pierre
Larmande
题目:Data Integration and
Knowledge Extraction in Agronomy: Examples & Perspectives
Exploring the links between genetic and
phenotypic traits is an important area of research in agronomy. One of the main
objectives of this is to accelerate the development of important traits that
can positively impact the agricultural economy. However, due to the existence
of complex molecular interactions, to gain complete understanding will warrant
data analyses performed at different molecular and environmental levels for a
given (plant) subject. For instance, to understand how rice genes involved in metabolism
or signaling of growth regulators control the rice panicle architecture. While
high-throughput technologies have played a key role in accelerating and
generating the much-needed data, these can only partially capture the dynamics
in genotype- phenotype relations. Consequently, our knowledge of the complex
relationships between the different molecular actors responsible for the
expression of the phenome in various plant systems remains fragmented. Hence,
there is an urgent need to effectively integrate and assimilate complementary
information to understand the biological system in its entirety.
We have developed AgroLD (www.agrold.org), a
knowledge graph system that exploits the Semantic Web technology and FAIR
principles [2], to integrate information to integrate data about plant species
of high interest for the plant science community e.g., rice, wheat, Arabidopsis
and in this way facilitating the formulation of new scientific hypotheses. We
present some integration results of the project, which currently focused on
genomics, proteomics and phenomics. AgroLD is now a Knowledge Graph database of
900M triples created by annotating and integrating more than 100 datasets
coming from 15 data sources –such as Ensembl plants, Gramene.org and TropGeneDB
– with 15 ontologies –such as the Gene Ontology and Plant Ontology. Our
objective is to offer a domain specific knowledge platform to solve complex
biological and agronomical questions related to the implication of genes in,
for instances, plant disease resistance or high yield traits. We expect the
resolution of these questions to facilitate the formulation of new scientific
hypotheses to be validated with a knowledge-oriented approach.
法国国家农业食品与环境研究院主任Claire Nedellec
题目:Making textual information and
experimental data interoperable in wheat breeding
We will show how automatic
information extraction from texts can be performed to obtain phenotypic and
genetic descriptions of wheat varieties. The diversity of text descriptions
makes the problem complex. We address it using text mining methods and
designing the Wheat Trait and Phenotype Ontology (WTO). We have designed the Taec open annotated corpus to evaluate and compare text mining
methods for this task. Textual information complements experimental phenotyping
data. To link them in the same knowledge management system, we develop a
knowledge model in which the classes of WTO are mapped to the classes of the ontology CO_321, dedicated
to wheat experiment indexing. Our formal framework contributed to the new SSOM mapping standard. Class mapping is made complex by the
diversity of labels and the purposes of use. Our alignment approach combines
lexical rules and expertise. Our final goal is to propose an unified RDF model
of ontologies, mappings, and data that will allow users to query heterogeneous
data in a powerful and seamless way.
AKIE2023 开幕倒计时
距6月10日正式开幕还有4天!
加入到我们,中国计算机领域最有活力与激情的群体当中来吧!
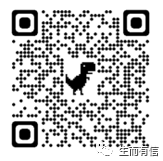
(扫码报名)